Our research focuses on understanding neural circuit principles that could ultimately inspire new solutions for difficult engineering problems. In particular, our research programs focus on understanding sensorimotor control algorithms in flying insects. Our approach is to get at a system-level understanding of a brain function by combining various experimental techniques such as electrophysiology, calcium imaging, optogenetics, and behavioral genetics. Another line of work in the lab involves developing computational models of the experimentally-derived neural algorithms, and thereby bringing new insights into engineering applications such as unmanned air vehicles (i.e., drones).
Specific research areas |
Recent results |
Higher-order Visuomotor Processing
We can almost effortlessly recognize others' faces from complex backgrounds and distinguish subtle differences in facial expressions. Such abilities can easily outperform state-of-the-art computer algorithms. How does a set of brain cells give rise to such a remarkable feat? We approach this question by studying the fruit fly's visual system. Owing to their premiere genetic toolkits, fruit flies have become one of the most popular model organisms in neuroscience. This tiny insect invests more than half of their nervous system into visual processing and exhibit a large repertoire of higher-order visuomotor functions, including object recognition/tracking, object avoidance, object distance estimation, selective attention, place learning, and gap width estimation. In our lab, we study how such visual features are represented in a neural circuit and triggers specific behavioral programs.
|
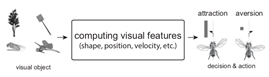
Park, H., Lee, J., Kim, A.J., Motion-independent visual computation underlies small object avoidance in flying Drosophila, CSHL Neurobiology of Drosophila meeting (2019), New York
|
Flight Control via Internal Predictions
Fruit flies are agile fliers. With wings that beat 200 times a second, they can hover, fly straight, avoid collision, turn rapidly, and even land on an improbable target, such as a ceiling. To make these sophisticated flight maneuvers, fruit flies constantly make use of their sensory feedback. In two previous studies (Cell 2017 and Nat Neurosci 2015), we demonstrated how a set of visual neurons guides fruit fly’s stabilization behaviors and how their visual signaling is quantitatively modulated by internal predictions to enable rapid flight turns. We aim to develop this study further by uncovering the detailed circuit mechanism of internal predictions.
|
Kim, A.J., Fenk, L.M., Lyu, C. and Maimon, G., 2017. Quantitative predictions orchestrate visual signaling in Drosophila. Cell, 168(1-2), pp.280-294.
|
Modeling Brain-inspired Predictive Control
Flies' flight abilities outperform any man-made flying device, in terms of their stability, adaptability, maneuverability, and robustness. For this reason, quantitative analyses of neural mechanisms underlying flies' flight control is likely to help developing new solutions for man-made robots. In particular, the forward model can predict sensory feedback caused by a self-generated action, and that prediction can be used to selectively pass or block the neural signals from the sensory periphery. During flight, such control mechanisms can momentarily switch off the powerful stabilization system and permit aggressive flight maneuvers. Currently, we are developing a computational model of this control mechanism to apply it to simple engineering systems, such as an inverted pendulum or an unmanned air vehicle (i.e., drone).
|
|